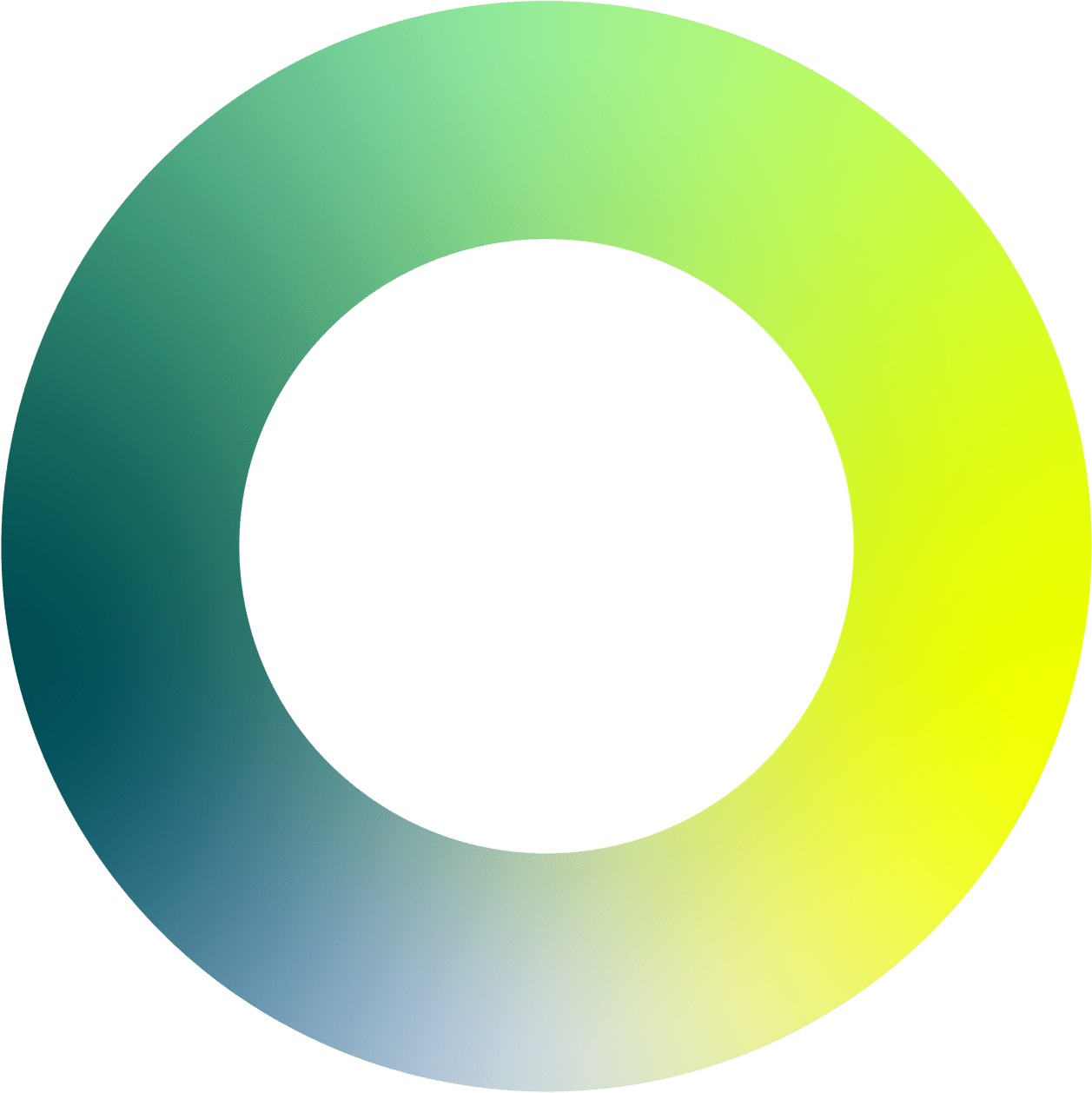
Member Resources
- Forgot My Password
- Change My Password
- My Details
- My Areas of Interest
- My Email Subscriptions
- My SIG Subscriptions
- My Event Registrations
- My Profile Image
- Company Contact Details
- Industries My Organisation Operates in
- SAP Solutions Used in My Organisation
- Document Directory
- Member Directory
- SAUG News
- Log Off